FinTech History
It’s been over 10 years since Prosper Marketplace started a non-bank lending platform in San Francisco. Since then the likes of LendingClub, SoFi, OnDeck, CommonBond have all emerged as the new cadre of marketplace lending or sometimes referred as P2P lenders. Credit Risk Models plays a central role in these new upstart Fintech lenders and today we will uncover some interesting tidbits around marketplace lender’s risk models.
Before we dive into credit risk modeling, let’s take a look at some of the latest trends and challenges of today’s Fintech marketplace lender. To start, “P2P” or “Peer to Peer” This is generally a misnomer description of the larger marketplace lender. In many ways, “marketplace” is somewhat of a misnomer for non-bank lenders. What’s common about these online lenders is the way they managed to by pass the need to use people’s deposits to lend like the traditional banks.
These lenders have linked up with hedge funds and wall street asset managers to lend directly to consumers. Fear not, the banks are still part of the equation of this new schema. Their role has been reduced to lending their federal banking charter for online lenders to lend across the US without adhering to State regulators and their usury laws.
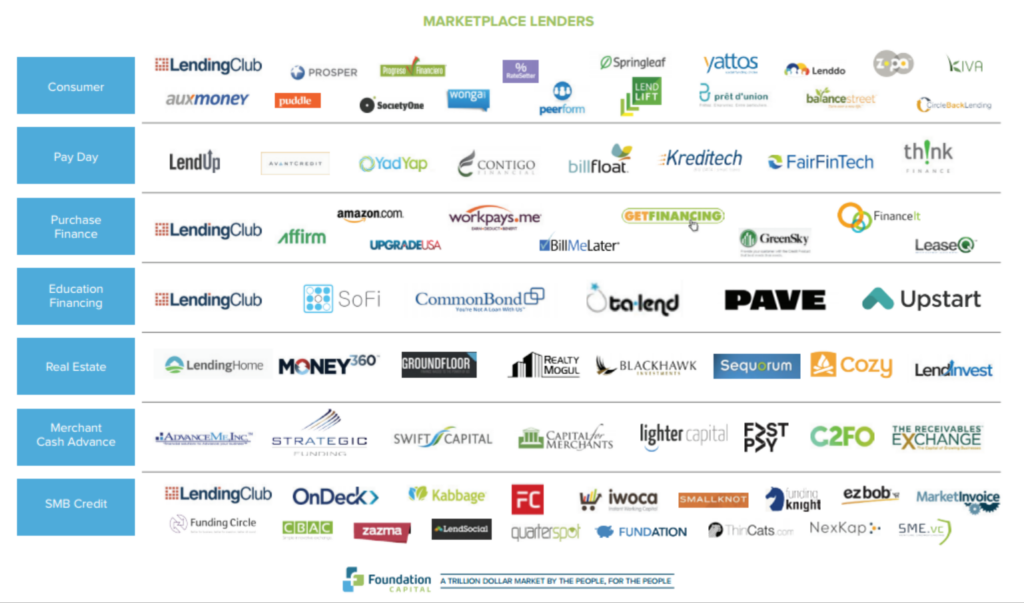
Challenges
There has been many court challenges as of late for this type of lending model, namely Madden vs. Midland. These court battles challenges the very essence of National Bank Act of 1863. The National Bank Act essentially allows federally chartered bank to override the state by state usury rates. Banks such as Chase, WellsFargo, Citi Bank amongst others are in essentially offering nation wide products without having to calibrate rates, fees and other characteristics of their product to each State.
Regardless of whether a bank, non-bank, p2p, marketplace lender is offering credit to the masses, each of these institutions regards a massive credit department to assess the credit worthiness of their perspective clients. In the old days, a branch manager will conduct an in person interview to the borrower. A series of qualitative and subjective questions are asked and extension of credit largely falls onto the experiences of the branch manager or the loan officer. In many cases, it is difficult to measure the quality of these decisions from one to the other and certainly many discriminatory acts prohibited nowadays probably managed to slip into that branch manager’s decision making process.
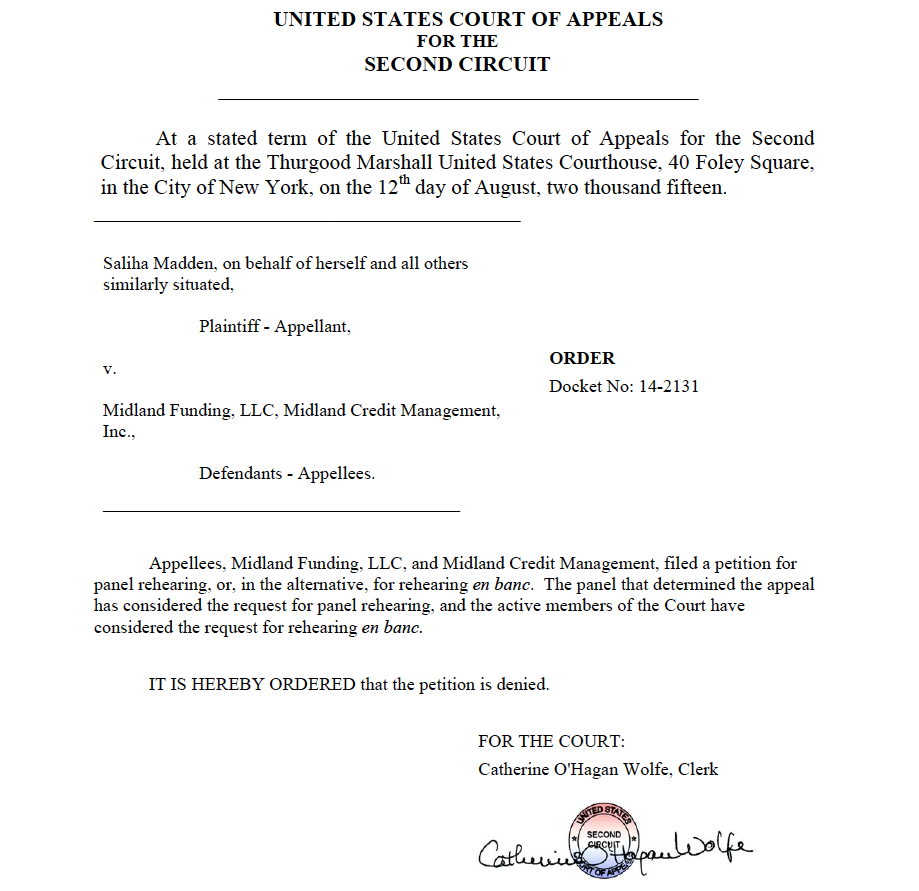
Risk Modeling Pitfalls
Today, with regulations such as Equal Credit Opportunity Act (ECOA), Fair Credit Reporting Act (FCRA), lenders must disclose and declare many aspects their underwriting process and system. Most lenders, especially banks will have to undergo extensive auditing requirements by the OCC or Office of the Comptroller of the Currency. Underwriting models and practices have come under heavy scrutiny by the newly established regulatory bodies such as CFPB, the Consumer Financial Protection Bureau.
Many credit risk or underwriting models have to be tested by third party audit firms for inadvertent discriminatory tendencies against protective classes such as race, ethnicity, national origin, age, gender or sexual orientation. Many lenders spend a ton of resources and energy to develop their in house credit risk model but only to find out later that their model is adversely impacting hispanics in certain areas. It is always a very expensive process to redesign the model in order to avoid hefty penalties later on.
Another credit risk modeling pitfalls is putting a score within a score. Often, if not careful, credit risk modelers always take the approach of baking in FICO or some other ready made credit bureau scores within it’s own credit risk model as documented here. There are many reasons why a custom model should not contain another scoring model for the simple reason that it would be impossible for anyone to explain the true predictive power of a credit model. In other words, no one truly knows the model attributes within FICO.
Credit Modeling Attributes
MaxDecisions Inc. has had many clients ask us to participate alongside their model development process to tease out these variables that may have an adverse effect down the line during the auditing phase. Our marketplace lender clients usually have a tendency to use variables such as renter vs. home owner. These variables look benign and innocent at first glance, however minorities more likely to be renters than home owners. Our client have probably the best intentions and the variable they have selected may have distinct predictive power, however they usually get caught up when a third party performs a model validation process. We have help many clients to avoid these pitfalls by using other variables that have similar predictive powers but much closer to a person’s credit worthiness than the ability to own a home due to their socioeconomic situation.
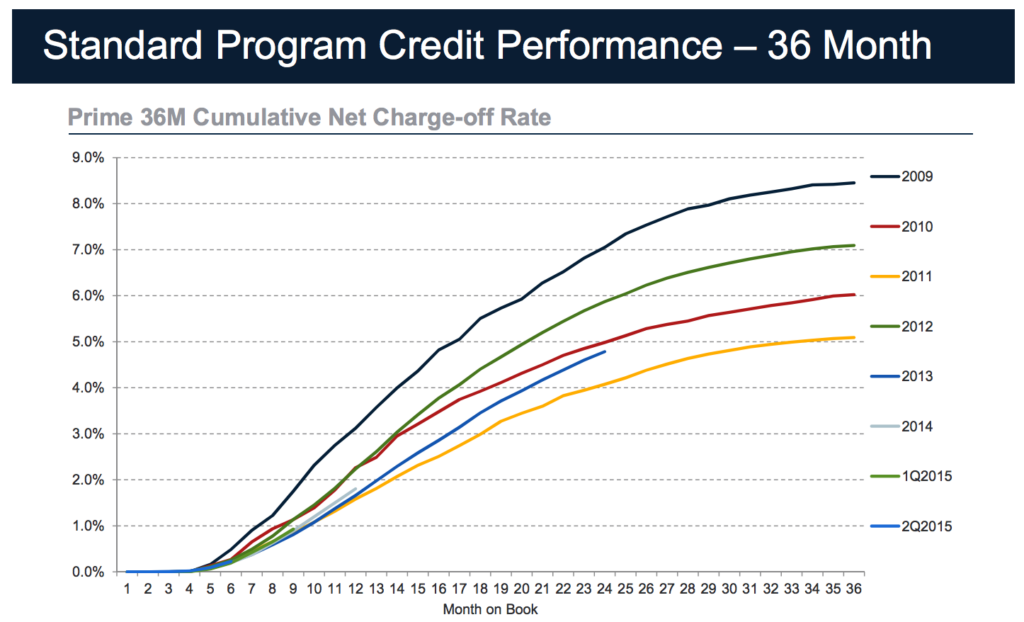
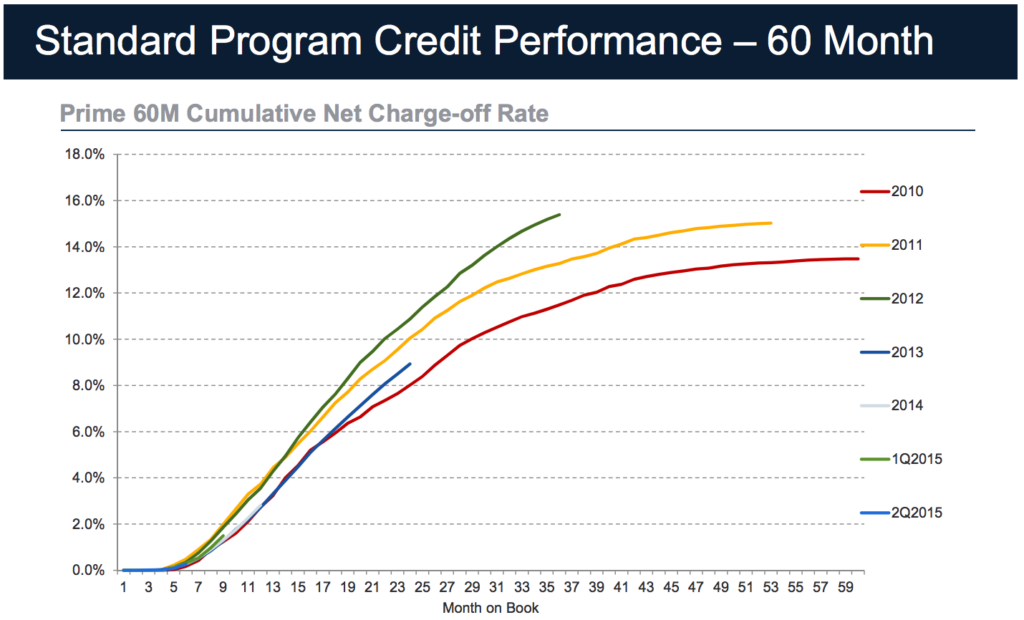
We often attempt to use Age and State as other model attributes that can be easily incorporated into a credit risk model. However, age is a protected class. It is against the law to discriminate or create inadvertent disparate impact against any protected classes such as age of an applicant. But not all is lost, there are other credit attributes that can be a substitute for age such as credit bureau record length that can act and predict as well as a person’s natural age. This derived variable may require a bit of processing before it can be used in your next credit risk model, but it’s well worth the reward for substituting a powerful predictive variable.
State or geography is another variable that we see clients use a lot in their modeling exercise. Yes, certain states carries higher credit risk than others but tagging Arizona, New Mexico as high risk will bound to be flagged during an model auditing process. However if you simply combine these states with other higher risk states together to create a dummy variable, the chances of your model getting called out as discriminatory might be lessened.
There are many cases of model attributes that can be switched to a more general variable to avoid regulatory and compliance risk without losing too much predictive power. You can always reach out to us for an opinion. We welcome all inquiries at info@maxdecision.com.
Until next time, happy modeling!